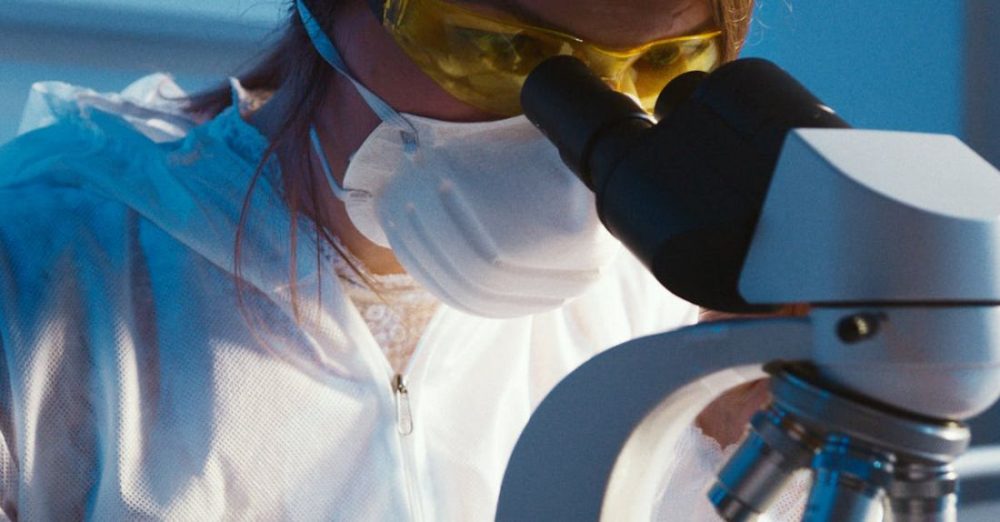
Machine learning has revolutionized numerous industries in recent years, and one field that has significantly benefited from this technology is bioinformatics. Bioinformatics involves the use of computational tools to analyze biological data, and the application of machine learning techniques has greatly enhanced our ability to make sense of the vast amounts of biological data generated through various experiments and studies. In this article, we will explore some of the key applications of machine learning in bioinformatics, highlighting how these technologies are driving innovation and discovery in the field of life sciences.
**Drug Discovery and Development**
One of the most impactful applications of machine learning in bioinformatics is in drug discovery and development. Traditional drug discovery processes are time-consuming and expensive, often requiring years of research and testing before a new drug can be brought to market. Machine learning algorithms can analyze large datasets of biological and chemical information to predict how different compounds will interact with biological targets, speeding up the process of identifying potential drug candidates. By leveraging machine learning, researchers can prioritize compounds for further testing, ultimately leading to faster and more efficient drug development processes.
**Genomics and Personalized Medicine**
Genomics, the study of an organism’s complete set of DNA, has been transformed by the advent of high-throughput sequencing technologies. Machine learning algorithms can analyze genomic data to identify patterns and variations associated with diseases, drug responses, and other biological traits. This information can be used to develop personalized treatment plans tailored to an individual’s genetic makeup, a field known as personalized medicine. Machine learning models can predict a patient’s response to different treatments based on their genetic profile, enabling healthcare providers to deliver more targeted and effective therapies.
**Protein Structure Prediction**
Understanding the three-dimensional structure of proteins is crucial for elucidating their functions and designing drugs that target specific protein interactions. Experimental methods for determining protein structures can be time-consuming and challenging, making computational approaches an attractive alternative. Machine learning algorithms can predict protein structures based on amino acid sequences, enabling researchers to quickly generate structural models for proteins of interest. These predictive models can provide valuable insights into protein function and interactions, guiding further experimental studies and drug discovery efforts.
**Biomarker Discovery**
Biomarkers are biological molecules or characteristics that can indicate the presence of a particular disease or physiological condition. Identifying relevant biomarkers is essential for early disease detection, monitoring treatment responses, and developing new diagnostic tools. Machine learning algorithms can analyze large-scale omics data, such as genomics, proteomics, and metabolomics, to identify potential biomarkers associated with specific diseases or conditions. By identifying biomarkers that are indicative of disease states, researchers can improve diagnostic accuracy and develop targeted therapies for better patient outcomes.
**Microbiome Analysis**
The human microbiome, the collection of microorganisms that inhabit our bodies, plays a critical role in human health and disease. Machine learning techniques have been instrumental in analyzing microbiome data to understand the complex interactions between microbial communities and their host. By integrating multi-omics data and clinical information, machine learning models can identify microbial signatures associated with various diseases, such as inflammatory bowel disease, obesity, and cancer. This knowledge can lead to the development of microbiome-based therapies and interventions to modulate the microbiome for improved health outcomes.
**Ethical Considerations and Future Directions**
As machine learning continues to advance in bioinformatics, ethical considerations surrounding data privacy, algorithm biases, and transparency become increasingly important. Researchers and policymakers must address these challenges to ensure the responsible and equitable use of machine learning technologies in the life sciences. Looking ahead, the integration of machine learning with other cutting-edge technologies, such as blockchain and quantum computing, holds great promise for further accelerating discoveries in bioinformatics and transforming the landscape of healthcare and medicine.
In conclusion, the applications of machine learning in bioinformatics are vast and diverse, offering new opportunities for innovation and discovery in the field of life sciences. From drug discovery and personalized medicine to protein structure prediction and microbiome analysis, machine learning is revolutionizing how we approach biological data analysis and interpretation. By harnessing the power of machine learning, researchers can unlock new insights into complex biological systems, ultimately leading to improved healthcare outcomes and a deeper understanding of life itself.