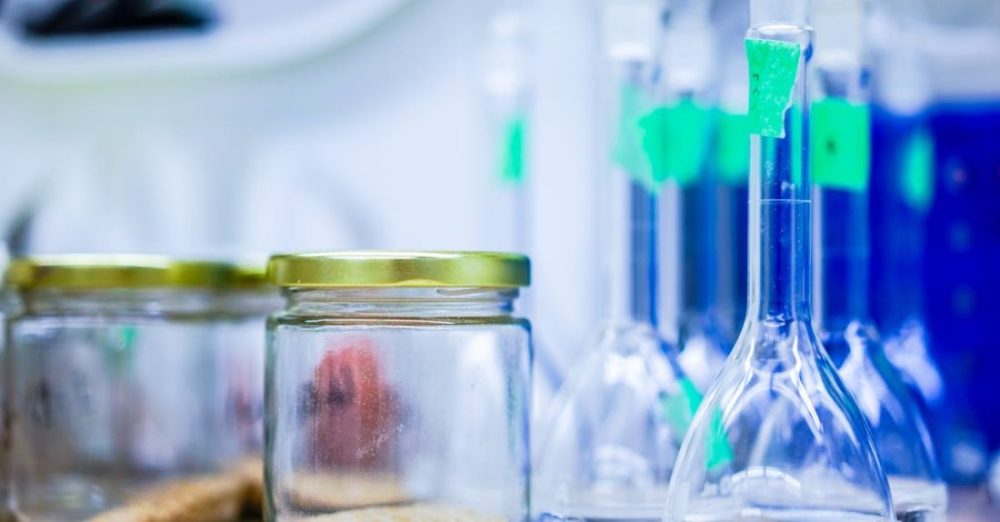
Computational biology is a fascinating field that blends biology, computer science, and mathematics to address complex biological questions through data analysis, modeling, and simulation. In this rapidly evolving discipline, researchers rely on a variety of key tools to process vast amounts of biological data, extract meaningful insights, and make predictions that can enhance our understanding of living systems. Let’s explore some of the essential tools that computational biologists use in their work.
**Sequence Alignment Tools**
One of the fundamental tasks in computational biology is comparing and aligning biological sequences, such as DNA, RNA, or protein sequences. Sequence alignment tools enable researchers to identify similarities and differences between sequences, which can reveal evolutionary relationships, functional motifs, and genetic variations. Popular tools like BLAST (Basic Local Alignment Search Tool) and Clustal Omega are widely used for sequence alignment due to their speed, accuracy, and user-friendly interfaces.
**Genome Assembly Software**
Genome assembly is the process of reconstructing complete genomes from fragmented DNA sequences obtained through high-throughput sequencing technologies. Genome assembly software plays a crucial role in piecing together these sequences like a jigsaw puzzle to create a comprehensive picture of an organism’s genetic makeup. Tools such as SPAdes, Velvet, and SOAPdenovo are commonly used for de novo genome assembly, facilitating the study of genetic diversity, gene expression, and evolutionary history.
**Phylogenetic Analysis Programs**
Phylogenetic analysis involves the study of evolutionary relationships among organisms based on their genetic or biochemical characteristics. Phylogenetic analysis programs help researchers construct phylogenetic trees that depict the evolutionary history and relatedness of different species. Tools like MEGA, PhyML, and MrBayes provide algorithms for inferring phylogenetic relationships, estimating divergence times, and visualizing evolutionary patterns, allowing scientists to explore biodiversity and evolutionary processes.
**Molecular Docking Software**
Molecular docking is a computational method used to predict the binding interactions between a small molecule (ligand) and a target protein (receptor). Molecular docking software allows researchers to explore potential drug candidates, study protein-ligand interactions, and design novel therapeutic compounds. Tools such as AutoDock, DOCK, and GOLD employ algorithms to simulate the docking process, predict binding affinities, and generate 3D models of protein-ligand complexes, aiding in drug discovery and development.
**Systems Biology Platforms**
Systems biology aims to understand biological systems as integrated networks of genes, proteins, and metabolites that function together to sustain life. Systems biology platforms integrate experimental data with computational models to analyze and simulate complex biological processes at a systems level. Platforms like COPASI, CellDesigner, and BioNetGen enable researchers to construct biochemical networks, perform dynamic simulations, and predict emergent properties of biological systems, facilitating the study of diseases, drug responses, and cellular functions.
**Machine Learning Algorithms**
Machine learning algorithms have become indispensable tools in computational biology for analyzing large-scale biological data, identifying patterns, and making predictions. By training algorithms on diverse datasets, researchers can develop models that classify gene expression patterns, predict protein structures, and uncover biomarkers for diseases. Algorithms such as Random Forest, Support Vector Machines, and Neural Networks are used to extract valuable insights from genomics, proteomics, and transcriptomics data, advancing personalized medicine and precision health initiatives.
**Conclusion:**
In conclusion, computational biology relies on a diverse array of tools and techniques to unravel the complexity of biological systems, from molecules to ecosystems. By harnessing the power of sequence alignment tools, genome assembly software, phylogenetic analysis programs, molecular docking software, systems biology platforms, and machine learning algorithms, researchers can decipher the mysteries of life with unprecedented precision and depth. As technology continues to advance and data generation accelerates, the role of computational biology in driving biological discovery and innovation will only grow in importance, shaping the future of biomedical research and biotechnology.