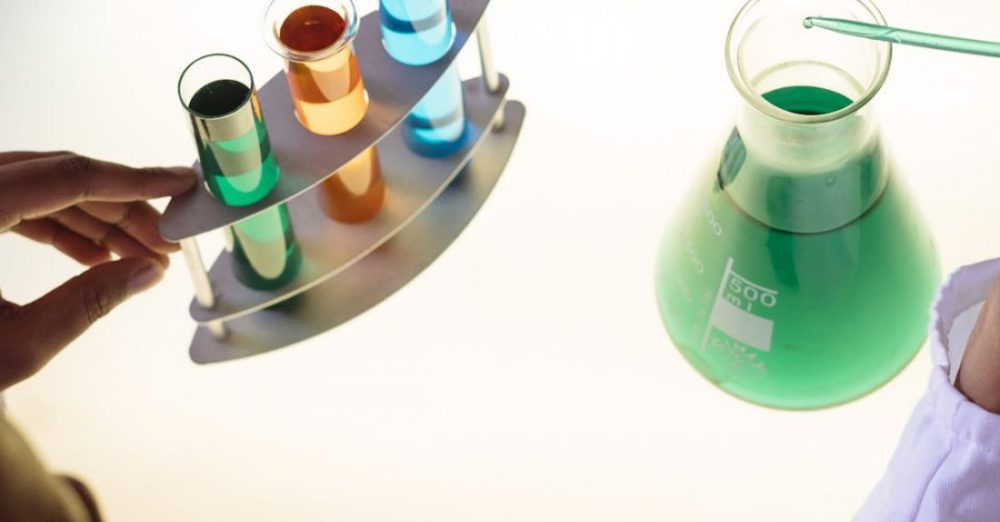
Computational biology, an interdisciplinary field that merges biology, mathematics, and computer science, has revolutionized biological research in recent years. By utilizing computational techniques to analyze and model biological data, researchers can gain valuable insights into complex biological processes. However, despite its numerous advantages, computational biology research comes with its fair share of challenges that scientists must overcome to make meaningful progress in the field.
Data Complexity and Size
One of the primary challenges in computational biology research is the sheer complexity and size of biological data. With advancements in technology, researchers can now generate vast amounts of data from various biological sources, including genomics, proteomics, and metabolomics. Analyzing and interpreting this massive volume of data requires sophisticated computational tools and algorithms. Moreover, integrating data from multiple sources to create comprehensive models of biological systems poses a significant challenge due to the diverse nature of biological data.
Algorithm Development
Another key challenge in computational biology research is the development of accurate and efficient algorithms for data analysis and modeling. Biological systems are incredibly complex, and traditional computational methods may not always be suitable for capturing the nuances of biological processes. Researchers must constantly innovate and develop novel algorithms that can handle the intricacies of biological data, such as non-linearity, noise, and uncertainty. Additionally, algorithms must be scalable to accommodate the increasing size of biological datasets and be able to process data in a timely manner to meet the demands of modern research.
Interdisciplinary Collaboration
Computational biology research requires close collaboration between biologists, mathematicians, computer scientists, and other experts from diverse fields. Effective interdisciplinary collaboration is essential for tackling complex biological problems that require expertise from multiple disciplines. However, coordinating efforts and communication between researchers from different backgrounds can be challenging due to differences in language, methodologies, and research goals. Building interdisciplinary teams that can work cohesively and leverage the strengths of each discipline is crucial for the success of computational biology research projects.
Validation and Reproducibility
Ensuring the validity and reproducibility of computational biology research findings is a significant challenge faced by researchers in the field. Validating computational models and predictions against experimental data is essential for establishing the credibility of computational approaches in biology. However, biological experiments are often subject to variability and noise, making it challenging to validate computational models accurately. Reproducing research findings and ensuring that results are consistent across different datasets and laboratories is another hurdle that researchers must overcome to establish the reliability of computational biology research.
Ethical and Privacy Concerns
As computational biology research delves into areas such as personalized medicine, genetic engineering, and biotechnology, ethical and privacy concerns become increasingly prominent. The use of genetic and personal data in research raises questions about data security, consent, and potential misuse of sensitive information. Researchers must navigate ethical considerations and adhere to strict data protection regulations to ensure that their work is conducted ethically and responsibly. Balancing the benefits of computational biology research with ethical and privacy considerations is a complex challenge that requires careful consideration and oversight.
Future Directions and Opportunities
Despite the challenges inherent in computational biology research, the field holds immense promise for advancing our understanding of complex biological systems and driving innovation in biomedicine and biotechnology. By addressing the challenges of data complexity, algorithm development, interdisciplinary collaboration, validation, and ethical considerations, researchers can harness the power of computational techniques to make significant contributions to the field of biology. Embracing emerging technologies such as artificial intelligence, machine learning, and big data analytics can open up new avenues for exploration and discovery in computational biology research.
In conclusion, computational biology research presents a unique set of challenges that require innovative solutions and collaborative efforts to overcome. By addressing the complexities of biological data, developing advanced algorithms, fostering interdisciplinary collaboration, ensuring validation and reproducibility, and addressing ethical considerations, researchers can unlock the full potential of computational biology to drive scientific progress and improve human health. Despite the obstacles that lie ahead, the future of computational biology research is bright, offering exciting opportunities for exploration and discovery at the intersection of biology and computational science.